AI can now model and design the genetic code for all domains of life with Evo 2
Arc Institute develops the largest AI model for biology to date in collaboration with NVIDIA, bringing together Stanford University, UC Berkeley, and UC San Francisco researchers
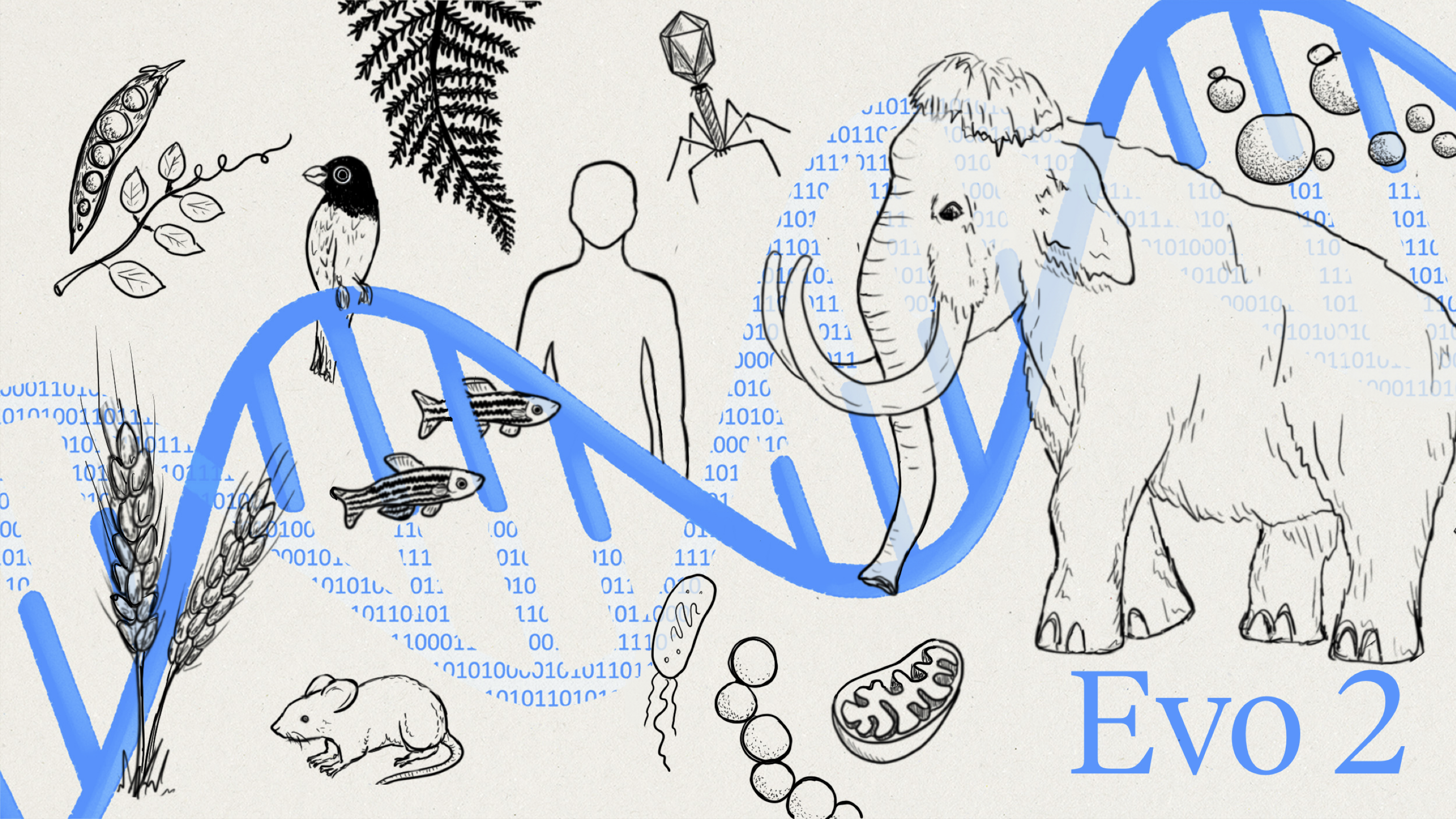
Arc Institute researchers have developed a machine learning model called Evo 2 that is trained on the DNA of over 100,000 species across the entire tree of life. Its deep understanding of biological code means that Evo 2 can identify patterns in gene sequences across disparate organisms that experimental researchers would need years to uncover. The model can accurately identify disease-causing mutations in human genes and is capable of designing new genomes that are as long as the genomes of simple bacteria.
Evo 2’s developers—made up of scientists from Arc Institute and NVIDIA, convening collaborators across Stanford University, UC Berkeley, and UC San Francisco—will post details about the model as a preprint on February 19, 2025, accompanied by a user-friendly interface called Evo Designer. The Evo 2 code is publicly accessible from Arc’s GitHub, and is also integrated into the NVIDIA BioNeMo framework, as part of a collaboration between Arc Institute and NVIDIA to accelerate scientific research. Arc Institute also worked with AI research lab Goodfire to develop a mechanistic interpretability visualizer that uncovers the key biological features and patterns the model learns to recognize in genomic sequences. The Evo team is sharing its training data, training and inference code, and model weights to release the largest-scale, fully open source AI model to date.
Building on its predecessor Evo 1, which was trained entirely on single-cell genomes, Evo 2 is the largest artificial intelligence model in biology to date, trained on over 9.3 trillion nucleotides—the building blocks that make up DNA or RNA—from over 128,000 whole genomes as well as metagenomic data. In addition to an expanded collection of bacterial, archaeal, and phage genomes, Evo 2 includes information from humans, plants, and other single-celled and multi-cellular species in the eukaryotic domain of life.
“Our development of Evo 1 and Evo 2 represents a key moment in the emerging field of generative biology, as the models have enabled machines to read, write, and think in the language of nucleotides,” says Patrick Hsu (@pdhsu), Arc Institute Co-Founder, Arc Core Investigator, an Assistant Professor of Bioengineering and Deb Faculty Fellow at University of California, Berkeley, and a co-senior author on the Evo 2 preprint. "Evo 2 has a generalist understanding of the tree of life that's useful for a multitude of tasks, from predicting disease-causing mutations to designing potential code for artificial life. We’re excited to see what the research community builds on top of these foundation models.”
Evolution has encoded biological information in DNA and RNA, creating patterns that Evo 2 can detect and utilize. “Just as the world has left its imprint on the language of the Internet used to train large language models, evolution has left its imprint on biological sequences,” says the preprint’s other co-senior author Brian Hie (@BrianHie), an Assistant Professor of Chemical Engineering at Stanford University, the Dieter Schwarz Foundation Stanford Data Science Faculty Fellow, and Arc Institute Innovation Investigator in Residence. “These patterns, refined over millions of years, contain signals about how molecules work and interact.”
Evo 2 was trained for several months on the NVIDIA DGX Cloud AI platform via AWS, utilizing over 2,000 NVIDIA H100 GPUs and bolstered by collaboration with NVIDIA researchers and engineers. The model can process genetic sequences of up to 1 million nucleotides at once, enabling it to understand relationships between distant parts of a genome. Achieving this technical feat required the research team to reimagine how an AI model could quickly ingest and make inferences about this scale of data. Greg Brockman (@gdb), Co-Founder and President of OpenAI, spent part of a sabbatical working to tackle this problem. The resulting AI architecture, called StripedHyena 2, enabled Evo 2 to be trained with 30 times more data than Evo 1 and reason over 8 times as many nucleotides at a time.
The model already shows enough versatility to identify genetic changes that affect protein function and organism fitness. For example, in tests with variants of the breast cancer-associated gene BRCA1, Evo 2 achieved over 90% accuracy in predicting which mutations are benign versus potentially pathogenic. Insights like this could save countless hours and research dollars needed to run cell or animal experiments, by finding genetic causes of human diseases and accelerating the development of new medicines.
In addition to genetic analysis, Evo 2 could be useful for engineering new biological tools or treatments. For example, “if you have a gene therapy that you want to turn on only in neurons to avoid side effects, or only in liver cells, you could design a genetic element that is only accessible in those specific cells,” says co-author and computational biologist Hani Goodarzi (@genophoria), an Arc Core Investigator and an Associate Professor of Biochemistry and Biophysics at the University of California, San Francisco. “This precise control could help develop more targeted treatments with fewer side effects.”
The research team envisions that more specific AI models could be built with Evo 2 as a foundation. “In a loose way, you can think of the model almost like an operating system kernel—you can have all of these different applications that are built on top of it,” says Arc’s Chief Technology Officer Dave Burke (@davey_burke), a co-author on the preprint. “From predicting how single DNA mutations affect a protein's function to designing genetic elements that behave differently in different cell types, as we continue to refine the model and researchers begin using it in creative ways, we expect to see beneficial uses for Evo 2 we haven't even imagined yet.”
In consideration of potential ethics and safety risks, the scientists excluded pathogens that infect humans and other complex organisms from Evo 2’s base data set, and ensured that the model would not return productive answers to queries about these pathogens. Co-author, Tina Hernandez-Boussard (@tmboussard), a Stanford Professor of Medicine, and her lab members, assisted the team to implement responsible development and deployment of this technology.
“Evo 2 has fundamentally advanced our understanding of biological systems,” says Anthony Costa (@anthonycosta), director of digital biology at NVIDIA. “By overcoming previous limitations in the scale of biological foundation models with a unique architecture and the largest integrated dataset of its kind, Evo 2 generalizes across more known biology than any other model to date — and by releasing these capabilities broadly, the Arc Institute has given scientists around the world a new partner in solving humanity’s most pressing health and disease challenges.”
Please visit BioRxiv to access the related preprint "Genome modeling and design across all domains of life with Evo 2" and the sister machine learning paper "Systems and Algorithms for Convolutional Multi-Hybrid Language Models at Scale."
###
The Arc Institute (@arcinstitute) is an independent nonprofit research organization located in Palo Alto, California, that aims to accelerate scientific progress and understand the root causes of complex diseases. Arc’s model gives scientists complete freedom to pursue curiosity-driven research agendas and fosters deep interdisciplinary collaboration.